Digital transformation is a term we've been hearing a lot over the past decade—and for good reason. Transforming the way we work by adopting more efficient processes that are interconnected across the digital landscape promises a host of benefits for those who get it right. But, as with any journey into new territory, there are obstacles and challenges to navigate along the way.
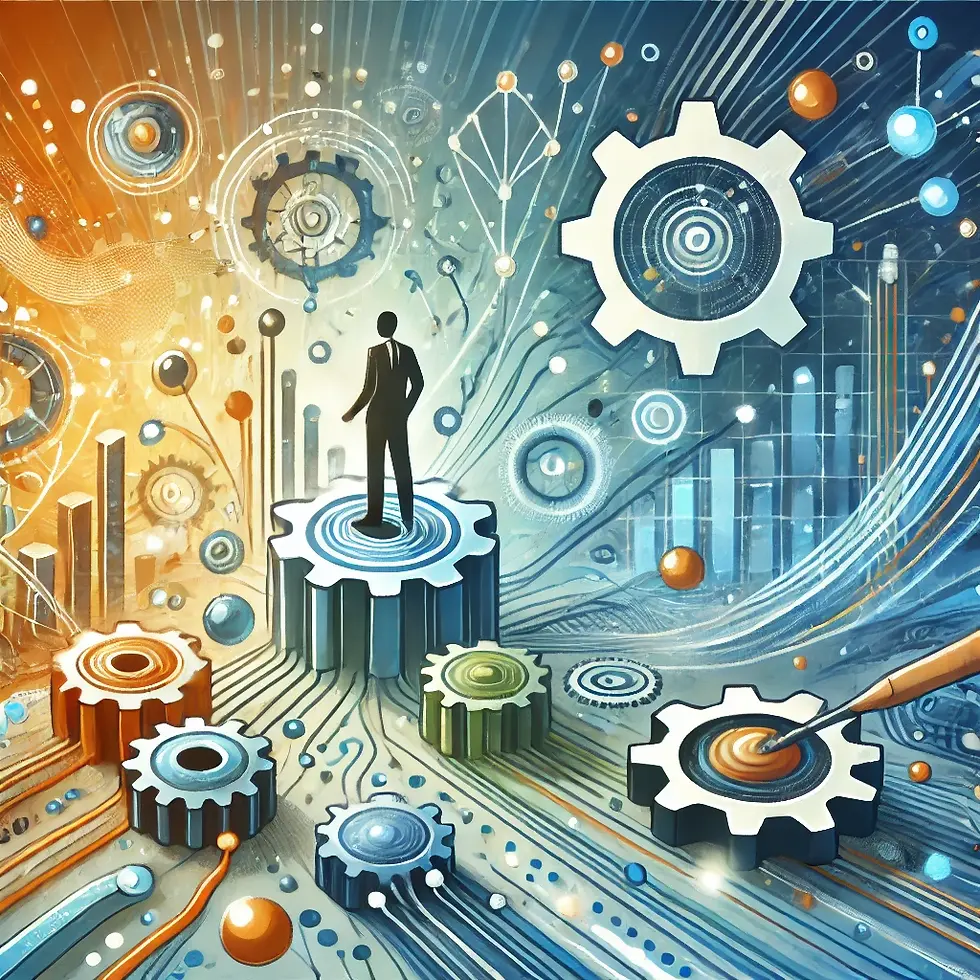
Interestingly, the largest issues are not typically about the technology—they're about the people. Resistance to change is natural and should be expected. Changing comfortable and well-established methods for something new is, for most, not an appealing prospect. But there are steps we can take to ease this transition. Clarity about the ‘What?’ and ‘Why?’ of the changes is a good start—ideally as part of a well-considered strategy with measurable objectives. Two-way communication with the team, especially those most impacted by the changes, is also critical. I've heard countless stories of companies pushing out changes, only to see them fall flat because some important factor or consideration was not understood earlier. Failed attempts are costly too—with each poorly implemented change, will and enthusiasm are spent.
I posted last week about planning for the Future of Work/Strategic Workforce Planning. I believe this area doesn’t get the attention it deserves. It's all well and good having a plan and a shiny new piece of kit, but you won't maximise your ROI if you haven’t managed skills and properly aligned roles.
Data and compute power seem to be the new economy. Ensuring data is clean, accessible, and secure is a big challenge, especially if you're weaving legacy systems and databases into a transformation project. I was speaking with a good friend recently about the importance of well-structured data, even when using advanced AI tools. It was interesting because there was an assumption that advanced AI models would be able to work through and sort out disorganised data. This is true, but only to a point. We need reliable data to build reliable models and avoid issues like AI hallucinations or false positives. The old adage of ‘rubbish in = rubbish out’ still holds true. But beyond this, data is a discipline—something to be governed and managed. The better this is done, the better the results.
There’s a lot of pressure to achieve results quickly. This is dangerous, as speed is often achieved at the expense of quality. It's similar to dieting: you can starve yourself for a week and lose a few kilos, but they'll immediately come back once you return to old habits. What's needed is well-considered, goal-oriented, measured, consistent change. This leads to long-term, sustainable improvement—even if the immediate results arrive more slowly.
How has your experience with transformation been? What measures do you put in place to ensure success?
Comentarios